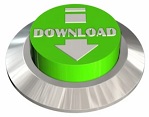
#CASCADE WINDOWS GRID PATTERNS KEYGEN#
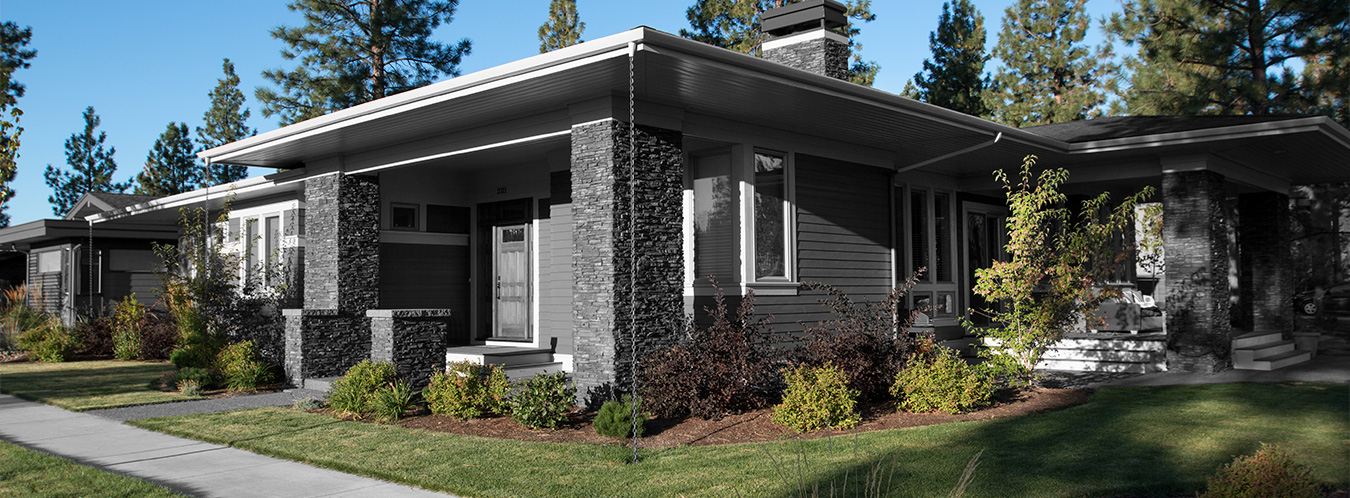
You, “ Data-driven prediction of unsteady flow over a circular cylinder using deep learning,” J. Khoo, “ Fast flow field prediction over airfoils using deep learning approach,” Phys. Sekar uses the convolutional neural network (CNN) and multi-layer perceptron (MLP) model to construct the mapping relationship between the geometric parameters, Reynolds number, and angle of attack of the airfoil and the parameters of the flow field around the airfoil. The proposed method is more effective than the original Spalart–Allmaras model. constructed a mapping relationship between the turbulent vortex viscosity and the average flow variable for the airfoil flow with high Reynolds numbers.
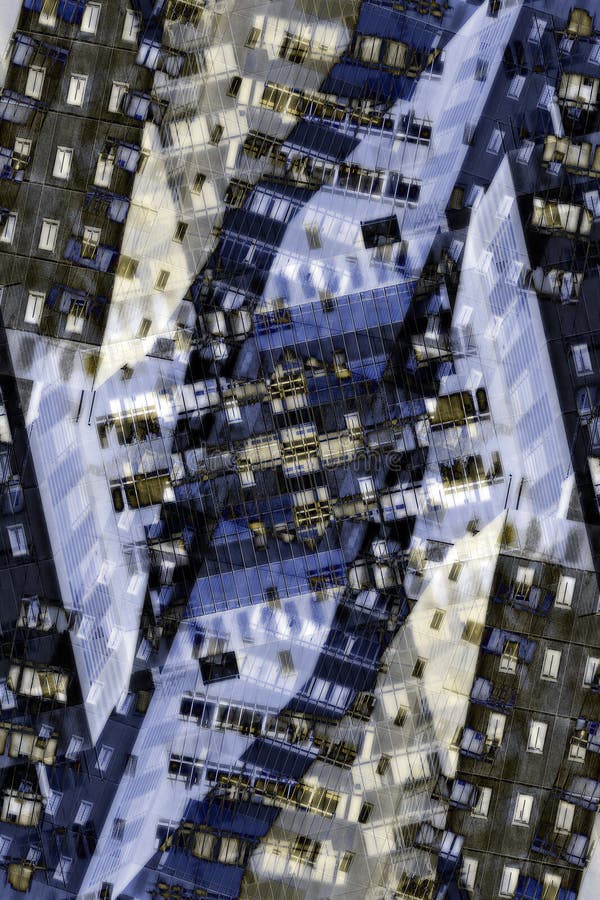
Liu, “ Machine learning methods for turbulence modeling in subsonic flows around airfoils,” Phys. uses the pressure distribution on the surface of a cylinder to predict the velocity field around the cylinder. Li, “ Prediction model of velocity field around circular cylinder over various Reynolds numbers by fusion convolutional neural networks based on pressure on the cylinder,” Phys. In this regard, many studies concerning the reconstruction of the flow field have recently emerged. To accurately reconstruct the characteristics of the flow field for accurate condition monitoring, a regression model needs to be constructed to reflect the mapping relationship between the turbulent variables. The application of the data-driven method to turbulence modeling can be divided into two categories, i.e., it provides improved closure of Reynolds stress, which provides an alternative method for modeling Reynolds stress, and it constructs a mapping relationship between turbulent variables instead of solving the original partial differential equation. Recently, data-driven techniques have been widely used for turbulence modeling. From another point of view, the recent success of deep learning provides a more effective data mining method for obtaining valuable deep-level internal mathematical laws from high-fidelity data. However, considering that numerical simulation is time consuming, more importantly, when the working state changes, the calculation needs to be performed again. Numerical simulation is widely used in engineering practice because it can simulate the flow state at a small cost. The results show that the worst linear correlation coefficient is 0.9848 in the testing set, indicating that the model has satisfactory ability to reconstruct and predict the flow field. Moreover, to accurately evaluate the regression model proposed by this study, the correlation analysis was also applied in this study.

In the testing set, the symmetrical model has a certain ability to realize extrapolation and prediction, and the flow field structure can be accurately reconstructed by using the discrete pressure values on the wall surface of the cascade channel. In order to verify the ability of the model to reconstruct unknown operating conditions, the organizational form of the training set and testing set has been specially designed to achieve the ability of interpolating outwards. The generalization ability and scalability of the model are analyzed from the contour plots of the pressure and density gradient. The symmetrical model includes a transposed convolution part and a conventional convolution part, which, respectively, implement up-sampling of the pressure data and further extraction of features. The dataset used for model training is derived from numerical simulation of the supersonic cascade channel. The model designed is to demonstrate that the deep neural network can realize the reconstruction and prediction of the flow field structure in the supersonic cascade channel under complicated and changing working conditions. A data-driven model containing a symmetrical deep neural network is proposed to reconstruct the flow field structure in a cascade channel by measuring discrete pressure values on the wall of the supersonic cascade channel.
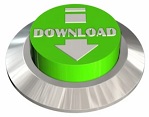